Privacy Economy I
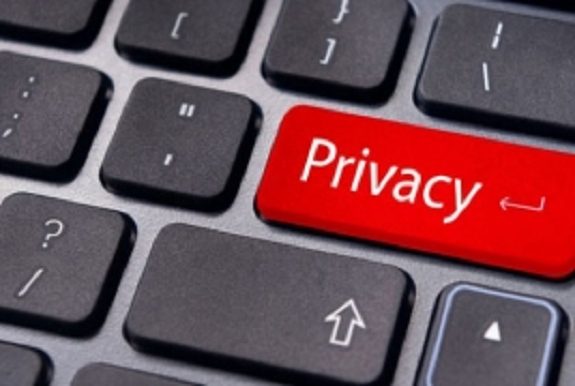
How to measure its value
Have you really thought about what
privacy is all about?
There is no formal description or legal definition as to human rights to privacy.
Politicians, philosophers and business have grappled with what privacy means when it comes to society and rules.
- Aristotle drew a distinction between public and private life (oikos).
- Schopenhauer pushed the idea of our intuitive ability to know things that are not formally stated.
- Karl Marx dismissed the idea of a private citizen as all belonged to the whole. Privacy and society at odds.
- The phone operates on a wireless carrier network that detects where you physically are,
- the fitness sensor shows your health status in short time increments,
- the car navigation system detects your mobility in space,
- the journey is identified by road sensor technology,
- the store performs facial recognition and tracks your movement as you move inside meanwhile,
- the home energy system senses that you have left on your journey.
Privacy is somewhat anachronistic to how species survive, humans being one example. In order to coexist and survive the principle of cooperation among members is fundamental to avoiding extermination. Early on, human ancestors formed groups depending on their habitat. From foraging, hunting, onto cultivation and animal husbandry, groups provided a more stable habitat compared with single individuals living apart. The concept of sharing came from assigning skills to the members who showed greater results and then sharing with others. Out of this evolved the concepts of storing things (inventory), exchanging things (barter), how to measure (arithmetics), how to value goods (scarcity and surplus) and how to manage this process for the benefit of society (economics.)
All the above required cooperation; otherwise building on the efforts of previous generations to improve the next proved difficult. Today, these principles operate in more complex ways. Still, measuring the value of interaction between privacy and societal good is not well described. This paper tries to measure value such that the delicate balance between privacy of the individual and society as a whole is maintained for the benefit of all.
The influence of Inventions
Historians observed how one societal group took advantage of another, usually by conflict but often by cooperation. Weaponry was an obvious benefit and still practiced. Cooperation at a higher level such as medicine, agriculture, and building techniques continued in parallel. In retrospect the continuous cycle of gaining advantage between competing societies evolved under the influence of invention and timing. Did members subjugate their individual aspirations on behalf of their society? Yes and no.
Often, individual privacy and collective privacy were not the same. Religious dictums played an important role as they were used on behalf of the body politic. Yet social memes grew organically from separate groups that organized into a single body, typically in a bounded geographical area of land. Within this structure invention was an edge to power and survival. Today, nothing has changed. So how do we measure individual and collective privacy?
Japan is a good example. Individuality is not a primary trait of its society. Belonging and conformity are prized as more valuable than individual actions. The collective view creates a set of rules by which individuals prosper and support the groups they belong to. Group privacy is more important. We are not talking of secret societies but groups that adhere to rules that are treated as more important than individual conduct. Collective privacy is about keeping rules to support the group. A group member gains more value from collective privacy at the expense of their own. In essence, this gives the group a competing edge against others, either by specific knowledge available only to the group or advantage by their relative size.
In more primitive or tribal societies, with smaller group sizes, privacy is not as powerful a group attribute. With fewer members, knowing about others is easier. Secrets are few. As groups morph into new ones, some secrets are kept helping the parent group maintain power. Some secrets migrate to the newer group to help it compete. Sound familiar?
Privacy as a Measure of Value
In today’s world of digital information accessible through Internet communication protocols, human activity is collected by third parties from endpoints and central data stores. By human activity we mean the corpus of identifiable attributes belonging to individuals and their various associations with others. Any device connected by electronic means can directly and indirectly produce a “fingerprint” of events. An event can be thought of as a digital transaction where metrics, attributes and resulting metadata is used to form an identity useful to third parties, with or without an individual’s consent. When coupled with government mandated data repositories, commercial databases and location aware systems, individual profiles are dynamically formed. With the massive growth in remote sensing and acquisition of big data in the “Internet of Things” (IoT), an individual’s privacy, however defined, becomes fragile.
A sample scenario best describes this issue. You turn on your smartphone, activate your fitness sensor, get into your car and drive to a store that tracks buyers by video and on-premise sensors. All this touches unrelated data acquisition systems that collectively can create a dynamic profile at any point in time:
In other words, you are directly and passively trackable. These examples are not futuristic. They exist today. Consider we are not talking about when and how you use any application on the phone as this is transactional data.
-Image courtesy of Shanghai Technology &Science Museum.
As we depend on Internet enabled digital devices, each provides a glimpse or slice of your behavior, creating a less opaque kaleidoscope of your identity. Unwittingly, one is a silent partner in behavioral targeting by others.
Behavioral Targeting is the catalyst for creating value in digital commerce: to connect, identify, engage and transact. It’s mostly driven by advertising but also by how people engage in searching for information, downloading and using applications. All this is measured by the triad of marketing techniques – to record events by recency, frequency and outcome.
- Image courtesy of authenticorganizations.com
Profiling is the first layer of identity which persists over time. The second layer measures relationships with others – the social identity across connected services. Belonging to groups is a key measure of individual behavior.
It drives identity formation that populates social networks and sentiments expressed by members along with their contribution to social dialog (posts, comments, tweets, profile and media sharing.) A third layer is best defined by the social
- Image courtesy of UbiVault.com
Measuring the value of profile targeting depends on which behavior is important in an event. In the example given, data flows have many recipients each with different focus. The value in any data flow is measured by its half-life, namely, how useful the data is per unit of time. The collective economic value is measured by which data attributes combine into different profile slices of an individual. The more invisible one becomes, the less collective data value.
The more private, the weaker the targeting.
Debates defining privacy were affected after 1960 by the development of legal privacy protection. This is when computers made it easier to transfer information from paper to digital storage. Some defended privacy focusing on control over personal information about oneself, while others proposed a broader concept of human dignity. Societies are in a constant struggle with maintaining a balance among groups of individuals that opt for change against others that prefer continuity. Knowing about who belongs to what and their makeup is useful to minimize disruption and future uncertainty.
This privacy equation is unbalanced. On one hand, governments want to know the makeup of their citizens. Surveillance in various forms is a powerful weapon to track someone without their consent. However, surveillance by citizens over organs of government and business is seen as a potential threat. Consider how smartphone cameras have leveled this balance in police actions, protest meetings, and so on. In 1998 David Brinn, in his book “Transparent Society”, argued the challenges of balancing the impact of technology on the fulcrum of privacy and freedom. Individuals have the right to “watch the watchers.”
To the point, what is privacy? Is it defined by the individual or society? If one tries to define it as a synonym (like) as opposed to its antonym (unlike), the result is a hodgepodge of ideas. Technology keeps interfering. To enable social stability, privacy is negotiable, hence it has value. As you reduce the friction of information, privacy is depleted. That’s what technology does, reducing the time to a decision. But there is no contract, social or commercial where the value of privacy is quantified.
Here is a real example, where FICO scores are used to determine credit worthiness of individuals and businesses in the US (full disclosure, the author helped design the system.) Is it a perfect measure? Of course not. Is it trusted? Only that business has accepted FICO as a short hand method to quickly decide whether to loan or sell an economic good. It is used to augment your profile from a financial sense impacting how you are treated in society. It’s a gateway that influences how you live. Was the individual party to this process? Perhaps by the choices they made in how they lived but not in a contractual sense where their information is treated as an asset.
Creating Value from Privacy
Let’s take a simple measure - your value based on your economic net worth. As you increase your worth, preventing others to take advantage is a concern. High net worth individuals are at risk more than people with less to lose. Fundamentally it applies to all. At a lower economic level, people are willing to give up more of their privacy in return for some benefit. This could be government economic support, or commercial treatment such as senior discounts and other rewards. You are willing to give up privacy in exchange for a benefit.
A commercial enterprise is willing to give some benefit in exchange to knowing something about the recipient, be it age, sex, location, family unit and more. Their motive is straightforward: what does it cost to acquire data on a prospect in exchange to providing the best price and terms? Whether this is driven by market share, competitive offerings, or brand loyalty, its about gathering data on customers and prospects. This cost is reflected by advertising, marketing and customer support.
We now have the first part of the value equation. What’s missing is the second part. How do you measure the price (cost) to acquire the data? If information has value, everyone tries to minimize to cost of getting the data, privacy be damned. But with enforced regulations such as the EU privacy initiative, these costs go up. Is the individual’s profile of value to the commercial enterprise? Of course. Does this value transfer directly to the consumer? Not always.
How value is transferred is numerous. Your membership in a supermarket rewards program creates significant discounts. They want you to shop with them. In return, they analyze your shopping habits and purchases to help them manage their product inventory and leverage their contract terms with suppliers. For airlines, mileage and frequency of travel triggers just in time offers and preferred treatment. With financial institutions, your net worth affords different levels of engagement. A pharmacy chain reports your prescriptions and shares that with drug companies.
This list of data profiling is endless. What’s missing is the engagement and notification of the individual. If their data is shared by others in the supply chain, why don’t people benefit for this non-transparent transfer of value? If they were part of the transaction, willingness to share further details on their “profile” would help all parties. It’s a simple redirection of costs for advertising and marketing spent on existing channels, to the benefit of the individual, but in a transparent and auditable way.
The Rise of Data Aggregators
You know the usual suspects - Facebook, Amazon, Apple, Alibaba, Google. Ecommerce aggregators collect and analyze data for their benefit and their partners. The second tier are businesses with a larger market share: Walmart, Target, United, Shell, WeChat, etc. The first group of suspects collects data, on behalf of their advertisers, the core of their revenue. The second group collects data on who they engage with, consumers, vendors, and advertisers. Together they are part of an information economy that uses purchase intent and transactions, in the physical world and online.
Their value proposition is how individual data can be harnessed at the most effective marginal cost to an information user. What we are seeing is a battle to acquire, model and offer information in a digital world. Unfortunately, this is happening invisible to the individual. The Internet is an untrusted environment. Data is exposed without our ability to examine and challenge its veracity. Data collected persists for a long time. A bad review for a restaurant is difficult to expunge if untrue. A product rating does the same thing when negative. As long as the “owner” of the original data is not part of an economic transaction, manipulation of “truth” is endemic. But suppose an individual willingly shares their kaleidoscopic profile for the benefit of a third party. The more accurate it is, the greater its value. How can this be done? It requires a formal definition of privacy data exchange.
- First, it’s immutable, an exchange occurred between parties, on a certain date, for a contracted price.
- Second, it’s transparent to the individual from which the information is transacted.
- Third, a mechanism for establishing value (its price) must exist.
- Fourth, a way for transferring value back to the individual must work without third parties.
- Fifth, this value must have a transfer equivalence between participants.
An Economic Model for the Value of Privacy
The economics boil down to a utility-surplus model. Attributes are organized into sets. Sets follow an ontology that creates formal definition of attribute properties. The W3C effort in semantic ontologies is one example. Within a set, attributes are statistically modeled for the appropriate end use (Z-scores, SVM, Conjoint Measures) Sets are input into a multi-dimensional probability matrix M-D exposed to third parties. Based on the score or measure reflected by the M-D value, a third party can use this information – but at a negotiated price.
Using the M-D measure leads either to a non-event, engagement, or transaction. These individual outcome metrics update a global anonymous M-D datastore as well as the individual’s M-D profile. Using machine learning algorithms, decision weights are updated in the global matrices. Over time, the collective use of measures update their values. This is important because an individual profile may have a value at T1 but later a lower or higher value at Tn.
How is this transferred value used by the Individual? Rather than demonstrating how it’s done today, there are new methods that could apply to a new economic model for privacy. Recall that immutability is a central requirement for information exchange. The ability to assign some “value” to the exchange is the second need. And, converting value into an equivalent good or service is necessary.
Blockchains and Tokens
The rise of alternative currencies such as Bitcoin and Ethereum have exposed the viability of blockchain methods for distributed, secure applications. Without going into details of how blockchains work, they support immutability and security of data without a centralized authority. A blockchain is the plumbing on which crypto currencies rely. In concert with another method – smart ledgers – they provide the mechanism to securely record transactions whose payload is defined by any application.
Now imagine all the data attributes that describe you, stored with you (at the edge), transformed into an M-D matrix of probabilities that measures your affinity across multiple categories. That is your profile, encrypted and not stored by any central entity. The same method of categorization that is used in your local M-D matrix is used in a central datastore, with one proviso. These are aggregated anonymously across many individuals. For someone who wants to query the central M-D matrices, they have the metric of how many individuals belong in the group (affinity.) What they don’t have is which individual belongs to the group. For that you need to a second level of privacy.
Proxy Agents
We connect in various ways through the Internet. At its core are a number of protocol layers and an addressing scheme – IPV4. Because this uses a 232 maximum address pool, an alternative method is rapidly gaining acceptance – IPV6 with 264 addresses, an infinite pool. This permits entities to reserve a block of such addresses for communication. In order to preserve a double-blind privacy wall between profile owner and M-D user, there needs to be a neutral agent or proxy to execute a transaction. For example, an advertiser (user) can send information to an individual based on their profile without knowing who they are. Even when the individual responds, say through a browser, the proxy agent is the only visible IP address to the requestor.
The proxy agents are transient in that they exist only for the transaction and are returned to the pool for the next request, without any history of the event.
Anyone trying to track the proxy will have no ability to link that agent to when it was reused by another individual with a different profile. No history, cookies, or other tracking beacons of any use hence the double-blind principle. The M-D repository collects profiles that have a hashed identifier. To reach an individual on behalf of an advertiser, they broadcast a request based on their M-D profile (push). If an individual responds the M-D repository only knows that an agent has transacted with one of their M-D profiles in their datastore, one of many, and has responded but not identifying the unique individual. There are additional steps that preserve anonymity, but the process does not provide information to the datastore about a specific individual.
The current ways of measuring impressions such as click throughs and engagement are unaffected, but privacy is maintained until a transaction is completed. What is different is that this approach lends itself to performing data modeling at the edge with the individual, and not at a central system. The central M-D datastore can be updated based on transaction outcomes without compromising an individual’s profile. One benefit is that it disintermediates the role of a data aggregator which relies on tracking online behavior across web sites and applications. With this approach privacy value is negotiated based on the individual intent or sentiment level at the time of the event. If the individual is just exploring, their value Is lower than if they have an intent to transact. This dynamic pricing method makes for an interesting case as there is an implied transfer of value to the individual to the user of their profile. It is analogous to lead generation pricing as compared with web site impression inventory pricing.
Summary
We explored what privacy means in a limited sense. We propose a claim that privacy can be measured, and a value established. Rather than changing the way businesses budget to advertise and market on the Internet, we offer the idea of reallocating existing budgets to engage the individual rather through data aggregators and web publishers. The idea of using proxy agents to preserve anonymity while helping businesses to find prospects is the primary goal. At the same time, ensuring privacy is paramount. Finally, we propose that companies explore the methods outlined to increase their response levels, improve their brand awareness and employ more sophisticated methods for behavioral targeting, but not at the expense of individual privacy.